Ethical considerations on synthetic data in everyday machine learning research for healthcare.
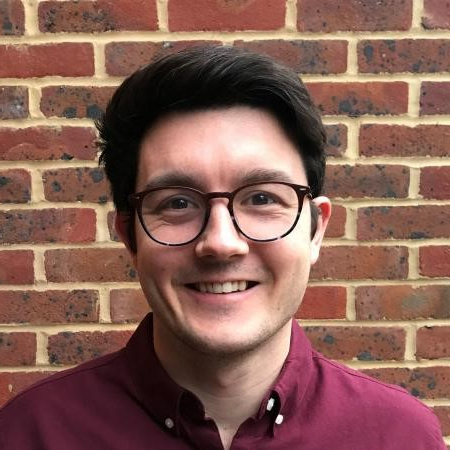
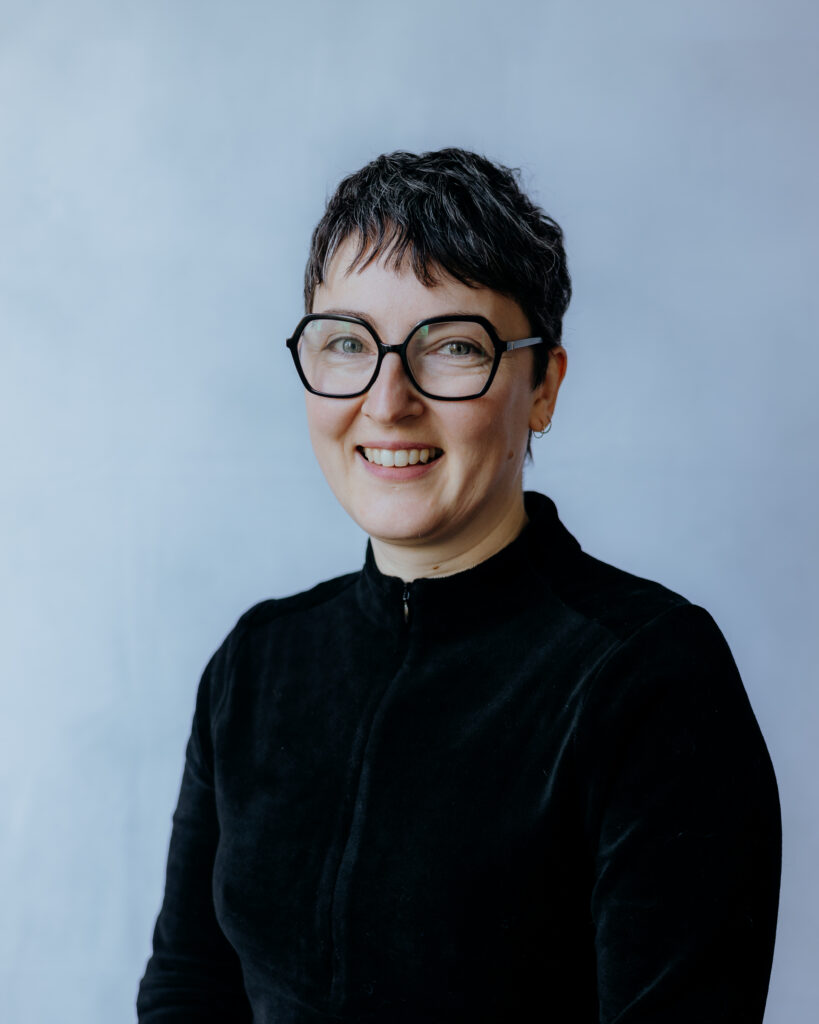
My hope is that synthetic data will significantly enhance machine learning models by unlocking new data sources and improving our understanding of their behaviour. My fear is that generating synthetic data may introduce errors and bias, potentially leading to inaccurate predictions with real-world consequences.
Dr Fergus Imrie, Florence Nightingale Bicentenary Fellow, University of Oxford.
As a postdoctoral research associate at KCESP, I lead the Creativity for Scientific Change Project, an initiative dedicated to broadening public participation in cutting-edge science in creative ways. Our mission is to bridge the gap between scientific discoveries and society by encouraging scientists to engage with diverse perspectives as they tackle the most complex ethical questions in rapidly evolving fields like Artificial Intelligence.
This two-part blog post features the 90-minute conversation I had with Dr Fergus Imrie, whom I had the chance to meet earlier this year. Dr Imrie was a Postdoctoral Fellow at the Van Der Schaar Lab and the New Cambridge Centre for AI in Medicine (CCAIM), and now he is a Florence Nightingale Bicentenary Fellow at the University of Oxford in the Department of Statistics.
In part one of this series (which you can read below in his blog post), Dr Imrie discusses his latest projects and reflects on the ethical challenges he encounters in his day-to-day research. In part two (which you can read by following this link or at the end of the page), I ask Dr Imrie how we can anticipate these ethical questions and his thoughts on creatively involving the public in addressing them.
Today, many people are familiar with GenAI technologies such as Open AI and Microsoft chatbot ChatGPT, either through personal use or from hearing about them in the news. However, GenAI encompasses more than just chatbots; it includes various forms of data generation that are much less known to the general public despite their significant implications for science and society.
Machine learning researchers can now create algorithms that identify and replicate patterns in existing medical datasets, generating ‘synthetic’ versions without needing to collect new data from real-world events, locations, or people. For example, instead of recruiting participants for a clinical study, researchers can extract data from existing patient records and model synthetic groups with specific parameters for health and disease.
This ability to generate realistic data opens new possibilities for medical research and machine learning technologies, but it also raises important ethical questions that require our collective careful attention.
Question: What kind of research are you involved in, and what projects have you been working on lately?
Answer: My research focuses on machine learning and artificial intelligence in medicine and healthcare. Recently, I’ve been working on two projects related to synthetic data. One project focused on creating synthetic data for a specific type of problem in healthcare called survival analysis. Survival analysis looks at whether or not a specific event will occur to an individual in the future. Let’s say our model looks at the incidence of cardiovascular disease, but an individual in the training dataset died of cancer after two years. We don’t know if this individual would have developed cardiovascular disease within 10 years because they, unfortunately, died from cancer. So, the model censored this individual at a two-year point to handle this missing information. To make up for this interesting aspect of the data, our question was: how can we better model this? Our solution was to generate new synthetic data for censored individuals.
In another project, we looked at using synthetic data to evaluate machine learning models in areas with very little data available, such as underrepresented or minority groups. We know that a model with only a few data points for a specific group may produce unreliable results. So, we generated new synthetic data to represent these small groups. We found that we could provide a significantly more accurate assessment of how well the model performs in these scenarios, especially with limited data about diverse populations. This is exciting because, up until now, people have mainly used synthetic data instead of private or sensitive data to train models. Instead, we have shown that we can now use synthetic data to probe a model in ways that were not possible before with existing real data. This is key to understanding the behaviour of machine learning models that was not apparent when using only real data.
Question: Could you share examples of the challenges and ethical considerations you have faced in your research?
Answer: There are many challenges with synthetic data. I would say that the fundamental question that most researchers think about is how to generate high-quality samples that are plausible and at the correct rate that you would observe in real data. There has been significant progress over the last ten years, with many researchers working on improving the quality and fidelity of synthetic data and models, particularly in fields like medicine.
There’s also a growing interest in privacy. Using generative models to create synthetic data can be risky if not done correctly. Models can copy the training data and generate new synthetic data from it without preserving privacy. This would be the worst kind of generative model as it would compromise the anonymity of the real data, resulting in the disclosure of real individuals and zero privacy being preserved. This issue is prevalent in the medical field and other areas, such as with generative models like ChatGPT, which can reproduce the exact text of a book without context only from a prompt. This leads to the different but related question: how can we distinguish between what’s real and synthetic? There are ongoing discussions in the field about the need to watermark AI-generated content.
But, when I think about ethics in my research, the key question is: Have we developed and implemented a model in the right way? Should the model be adjusted to address ethical considerations emerging from the problem we are trying to solve? I don’t believe any machine learning algorithm is intrinsically or inherently moral or non-ethical. It’s more about the broader process or system and the goal of the model and how it is deployed and used that determines its ethical implications. Suppose we introduce a new model and replace an existing way of estimating the risk of disease. We must be confident that it will benefit the population we are applying it to as a whole. But also, we must ask more specifically for which individuals will this model be better and worse? If the new model is worse for some people, should it be approved? How do we think about its worth? If the model underperforms, can we fix it, or do we need a different strategy using existing approaches for those people for whom the model is worse and the model for those for which the model is better? This then runs us into other questions on fairness: is it fair that some people get a better model and others don’t? Should everyone have the same model?
My research is on the more theoretical side of machine learning. When publishing a paper, we need to be prepared for the possibility of it being applied. However, the bar gets higher the closer a model gets to direct application, and there will be other stages in R&D to explore and mitigate this kind of ethical question. In the medical projects I’ve worked on, I haven’t encountered many concerns. Doctors and clinical practitioners have always been involved, and I have always felt comfortable raising genuine concerns about the project or discussing any questions I have encountered. When new technology is introduced into a field dealing with moral problems for a long time, it brings about different considerations. It raises various questions and issues that need to be addressed. But when I found something strange, I could speak to them and ask: OK, something weird is going on for this group of people. What might it be? Is this an issue? They have received training in medical ethics and help us follow ethical practices, guidelines, and existing literature.