Ethical considerations on synthetic data in everyday machine learning research for healthcare.
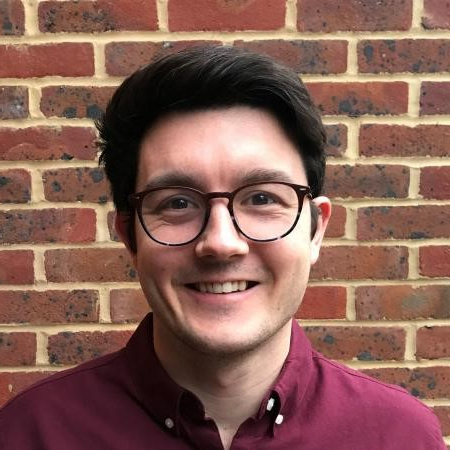
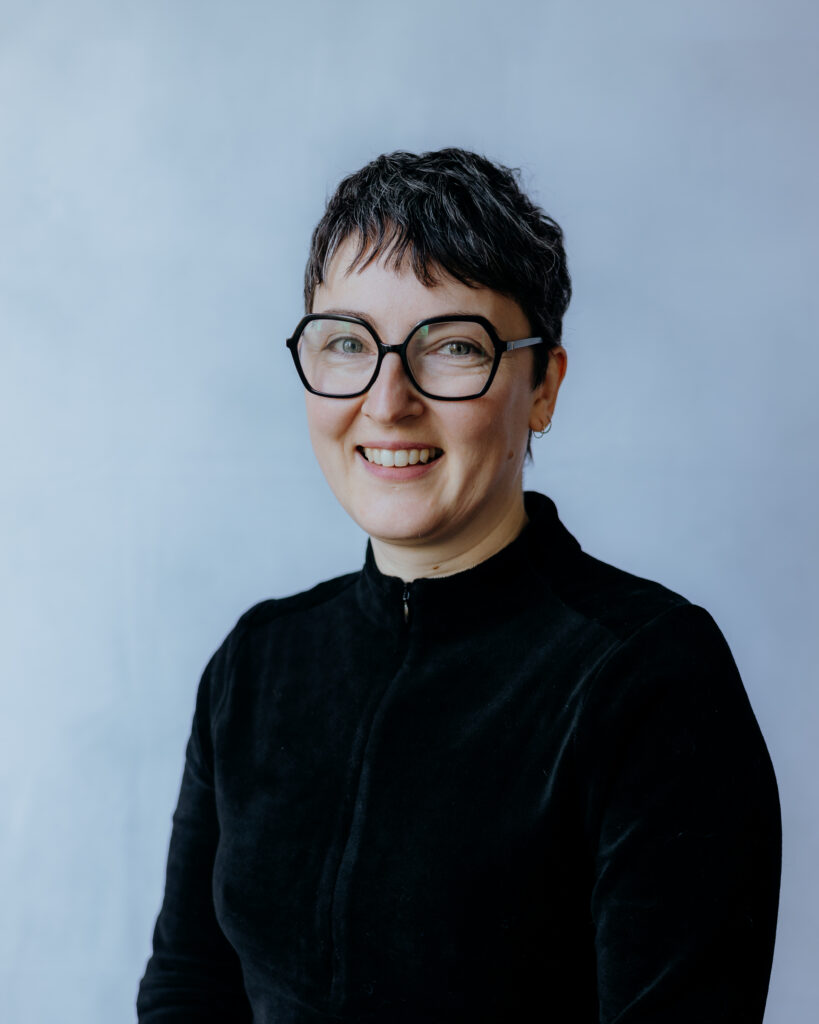
My hope is that synthetic data will significantly enhance machine learning models by unlocking new data sources and improving our understanding of their behaviour. My fear is that generating synthetic data may introduce errors and bias, potentially leading to inaccurate predictions with real-world consequences.
Dr Fergus Imrie, Florence Nightingale Bicentenary Fellow, University of Oxford.
Welcome to part two of our conversation with Dr Fergus Imrie, Florence Nightingale Bicentenary Fellow at the University of Oxford in the Department of Statistics.
In Part One of this interview (which you can read here), Dr Imrie discussed his research on GenAI and synthetic data in medicine and his daily ethical challenges, such as patients’ privacy and anonymity in LLMs, the dilemma of distinguishing between real and synthetic data, and the numerous questions on fairness that emerge from the development and use of medical models in healthcare.
In Part Two, we take the conversation a step further, exploring the complexities of anticipating ethical questions in GenAI research and examining how creative approaches could empower the public to actively participate in shaping the direction of future research.
Question: Have you thought about adding *ethical checkpoints* at key stages of model development to anticipate key questions before the models are used?
Answer: That’s a great question. I think ethics has only recently become a focus in machine learning, especially among researchers not involved in applied research, and it’s definitely still a work in progress. Some researchers working on the more theoretical spectrum of machine learning research have begun to think about ethics because they are concerned that papers could be evaluated based on ethics statements submitted to conferences. Still, most researchers typically don’t focus on the ethical implications of their research. Much research is presented as minor modifications or improvements to existing machine learning models, which leads to the question: if we have a technology that can perform a task without raising any ethical questions, and then we develop a new technology that can do the same task, just a little better, are there new potential ethical questions that weren’t previously a consideration? While there are valid arguments for and against this perspective, I believe it largely represents the majority of machine learning researchers, as evidenced by the ethics statements included in many publications.
In some cases, there is an almost emergent behaviour that arises when something becomes good enough to enable a new capability. Just because we can do something slightly better might not mean much until suddenly it does. For example, in 2014, researchers published a paper on a method for generating synthetic data called Generative Adversarial Networks (GANs). Many people were excited about this, as it was very different from the then-existing probabilistic methods. However, it wasn’t an immediate step-change in capabilities and I don’t recall any specific ethical concerns being raised. Over time, researchers improved and strengthened this approach to such an extent that researchers and the wider community began considering its potential applications and use cases, and ethical questions started to emerge. And now, we might even have very different ethical considerations at play on privacy, for example, depending on the settings or contexts it is applied to. Large Language Models (LLMs) are a great example. There were years of research and several clear precursors to today’s models used in academic tasks until ChatGPT was released, allowing everyone to interact directly with it. This was perceived as a step-change that brought a whole new set of ethical questions, such as the one I explained before on privacy and content generation, that need to be addressed immediately.
But don’t get me wrong, it’s not that these questions have suddenly appeared out of nowhere. In the example we were discussing, people have been talking about the Turing test, one of the oldest tests in computer science, based on whether a computer can fool a human into thinking it’s human. It has been shown that today’s models can do this in some limited contexts. Thankfully, for now, it is still possible to tell most of the time, but not always, and the fact that this debate is even happening is very exciting from a technical standpoint. But people have been discussing the issue of distinguishing between real and fake content, which is something that people have thought about before the release of ChatGPT. The impact of this technology is now much greater because more people are aware of it and are talking about it.
Question: Do you believe it’s important to discuss ethical questions with the public as a scientist, and how can creative practices help facilitate these discussions?
Answer: I think it’s important for the scientific and academic community to engage with the public, although it is far from commonplace. Maybe more people ought to be made more aware that it is an integral part of their job and to look for opportunities, but generally speaking, yes, definitely, this is part of me and my colleagues’ jobs.
As an AI researcher, I worry that many mainstream films and media depictions of AI are sensationalised. Often, the portrayal of AI is that it will either transform everything for the better or, on the flip side, the AI over-mind is coming. Obviously, it is more entertaining to present it in this way, but AI isn’t as good or as bad as they say. Actually, we have circled back to the start when we were talking about ethics. Rather than a textbook definition, I now think we all influence ethics, which varies depending on the questions and how they are asked. One could say, ok, you, scientists, go and talk to people about the concerns that they may have about, let’s say synthetic data. But people may not answer that direct question. It was very interesting to see how some of the artworks on AI and medicine we saw together were neither of those two things, but they opened up ethical questions in subtle and intriguing ways. They didn’t ask people questions directly, preparing them to answer difficult questions.
Thinking about synthetic data. What’s the best way of doing this? Does it have to be traditional, or can we be creative? We could show examples of the data that’s been generated, without the input and with the input, so that you can show how it is being enriched. Or we could show it as a chatbot-like system to have people interact and use it. This is one of the best ways of engaging because they can see the impact. They can directly experience versus simply being shown. Then, we could also think about synthetic data in a more medical context. But I guess it’s harder to show a dataset of 10,000 synthetic patients without trying to show the implications or how it somehow matches what we had before or how it preserves. I’ll keep this in mind when engaging with the public about my research in the future.
As GenAI and synthetic data research rapidly evolve, a thoughtful, collective response to their ethical challenges is crucial. Involving the public in these discussions is not just beneficial—it’s essential. Stay tuned for updates from the CSC project as we work to foster creative ways for the public to engage with these issues, ensuring diverse perspectives are reflected in cutting-edge AI research.
A special thanks to Dr Imrie for working with us to explore how ethical questions emerge during scientific practices. The future of GenAI and synthetic data is still being written, and we all have a role in ensuring it is developed responsibly for the benefit of society.